Theoretical computational chemistry was first developed in the 1970s. In 1998, this community was with the Nobel Prize to affirm its profound influence on quantum chemistry. The ability to describe real chemical reactions has been greatly advanced by hybrid algorithms combining classical and quantum mechanics. In 2013, the theoretical computational chemistry was again awarded with the Nobel Prize. Chemical events that appear in the virtual numerical world become the important information useful in contemporary development of chemical process technology.
For half a century, human society has burned fossil fuels to obtain energy, leading to ever-increasing greenhouse gases. This has become a problem that must be overcome if a global sustainable economy is to be maintained. Energy generated through solar power, combined with advanced catalytic electrode materials, carbon dioxide, and water, can produce electrolysis, converting energy into hydrocarbon fuel and oxygen. This is one recognized solution in the development of energy technology.
Hybrid algorithms mixed with classical and quantum mechanics can be employed in computer models to analyze the interaction of carbon dioxide and water molecules on the surfaces of electrode materials, and summarize the various chemical bonding or breaking events that occur during molecular conversion. Catalyst materials can be modified and redesigned to eliminate difficult reaction steps. These methods can be employed to conduct systematic comparative analyses in the virtual world, providing effective improvements in the actual chemical process and reducing both exploration/discovery time and development costs. The significant amount of digital data accumulated, coupled with the statistical methods of big data analysis, has resulted in novel and promising material designs, ushering in the next generation of experimental synthesis. Research and development models that effectively combine chemical processes and computational analyses will soon be mainstream, leading to future technological progress.
The concentration of carbon dioxide in the atmosphere continues to rise, and as a result, humans are facing global challenges such as global warming, extreme weather, rising sea levels, ecological crises, ocean acidification, etc. Therefore, the carbon dioxide in exhaust gas must be converted into useful chemical substances. It is essential that this problem be solved. One successful example is when natural photosynthesis occurs in plants; the carboxylated enzyme RuBisCO absorbs CO2 molecules, synthesizing new multi-carbon hydrocarbons in the Calvin cycle. In the chemical industry, scientists have actively developed various carbon dioxide conversion methods. In the past literature, metal electrodes have been used to carry out electrocatalytic reactions to reduce carbon dioxide in aqueous solutions. After comparing many metal electrodes (e.g., gold, silver, zinc, palladium, gallium, lead, mercury, indium, tin, cadmium, thallium, nickel, iron, platinum, titanium), researchers found that only copper electrodes could directly generate multi-carbon hydrocarbons. Full use of solar power should be considered as a means of generating electricity and developing appropriate catalytic electrodes to convert electrical energy, carbon dioxide, and water through electrocatalysis. The product could then be stored as chemical energy, significantly improving the efficiency of renewable energy.
In 2018, Professor Tsai’s team simulated the formation of OCCO on the surface of three copper oxides (i.e., CuO, Cu4O3, and Cu2O). The team first theoretically proposed that OCCO could be effectively synthesized on the surface of Cu4O3, in the presence of surface defects. The team’s conclusions were published in the PCCP journal of the Royal Society of Chemistry. In 2019, the German Siemens lab repeated the predictions made by the National Taiwan Normal University team, successfully producing the electrode catalyst Cu4O3 and converting CO2 into ethylene (C2H4).
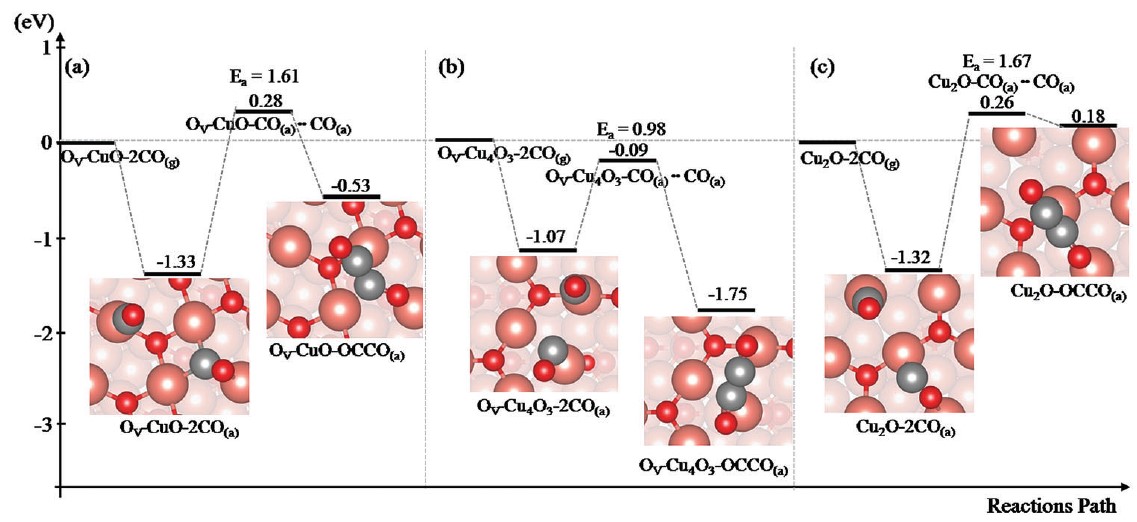
Figure 1: Potential energy surface prediction.
OCCO, an unstable intermediate, has a long molecular structure. In 2019, Professor Tsai’s team used its self-developed classical quantum hybrid simulation method to adjust the lattice structure of the copper electrode material to shape a long structure surface suitable for OCCO, further predicting that the long adjusted crystal lattice might be more suitable for synthesizing three-carbon hydrocarbons. Their research was published in the PCCP journal that same year.
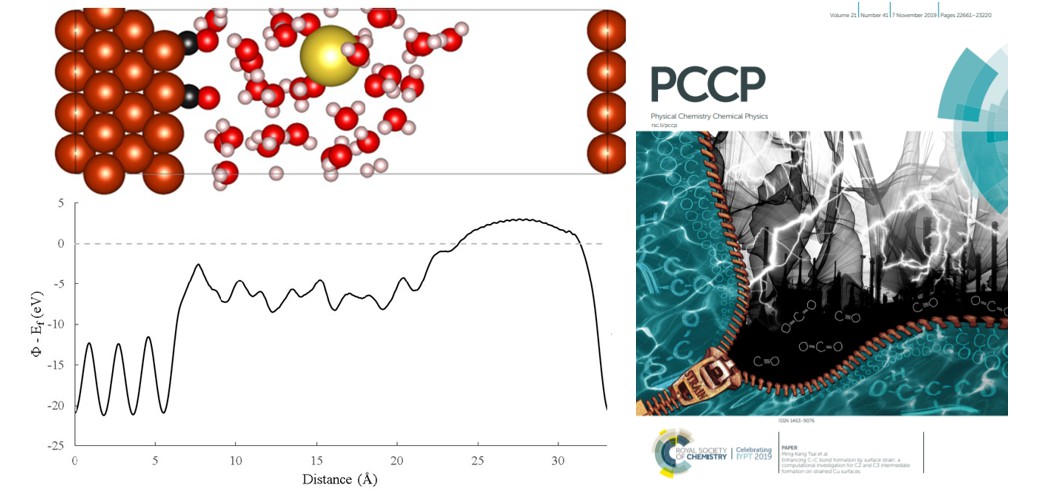
Figure 2: Hybrid model of CO electrocatalytic coupling on copper electrode surface.
In addition to the reduction of carbon dioxide, Professor Tsai's team has collaborated with Professor Chen Hao Ming's lab at the National Taiwan University to analyze iron-cobalt composite electrodes, exploring how to reduce the difficulty of splitting water molecules (a key step in hydrogen energy generation). Through live X-ray spectrum analysis combined with computer simulations of quantum chemistry, the team identified the roles of iron and cobalt on the surface of the electrode, successfully fabricating and analyzing the high electrocatalytic activity of the iron-cobalt composite electrode. The research results were published by the American Chemical Society in 2018.
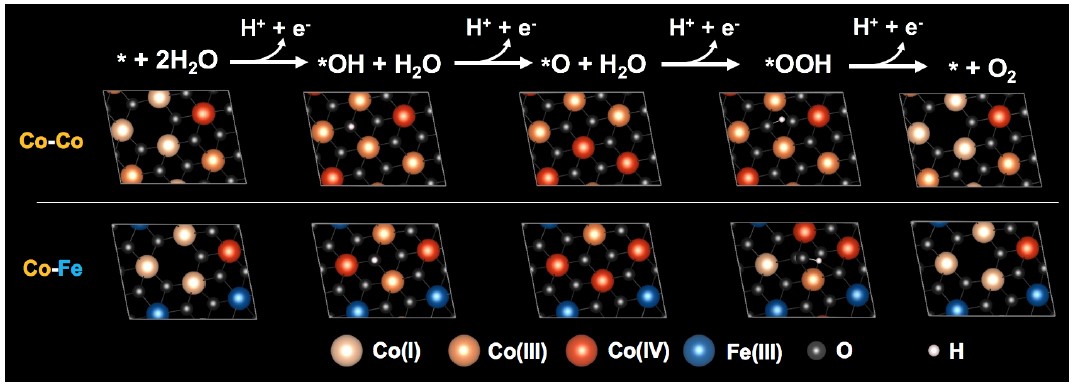
Figure 3: Schematic diagram of the reaction path of water oxidation on the surface of cobalt and iron-cobalt composite electrodes.
Theoretical computational chemistry can provide many opportunities for exploration that cannot be attempted in experimental conditions. By combining physical theory, programming, and chemical reaction principles, as well as the computing power of high-speed computers (for example, what is available from the National High-Speed Network and Computing Center, using the National Taiwan Normal University’s cloud computing platform), potential chemical reaction conditions can be predicted. Professor Tsai believes that the successful integration of theoretical computational chemistry and actual processing of experiments will become key factors in the advancement of renewable energy technology.
Reference
- "Identification of Stabilizing High-valent Active Sites by Operando High-energy Resolution Fluorescence-detected X-ray Absorption Spectroscopy for High Efficient Water Oxidation", Sung-Fu Hung,+ Yu-Te Chan,+ Chun-Chih Chang, Ming-Kang Tsai,* Yen-Fa Liao, Nozomu Hiraoka, Chia-Shuo Hsu, Hao Ming Chen,*J. Am. Chem. Soc.2018, 140, 17263-17270. Link: https://doi.org/10.1021/jacs.8b10722
- "A Computational Exploration on CO2 Reduction via CO Dimerization on Mixed-Valence Copper Oxide Surface", Chun-Chih Chang, Elise Y. Li,* Ming-Kang Tsai,* Phys. Chem. Chem. Phys.2018, 20, 16906-16909. Link: https://doi.org/10.1039/C8CP00592C
- "Enhancing C-C Bond Formation by the Surface Strain: Investigating the C2 and C3 Intermediate Formation on the Strained Cu Surfaces", Yu-Te Chan, I-Shou Huang, Ming-Kang Tsai,*Phys. Chem. Chem. Phys.2019, 21, 22704-22710. Link: https://doi.org/10.1039/C9CP02977J